The Importance of Bounding Boxes in Data Annotation for Business Success
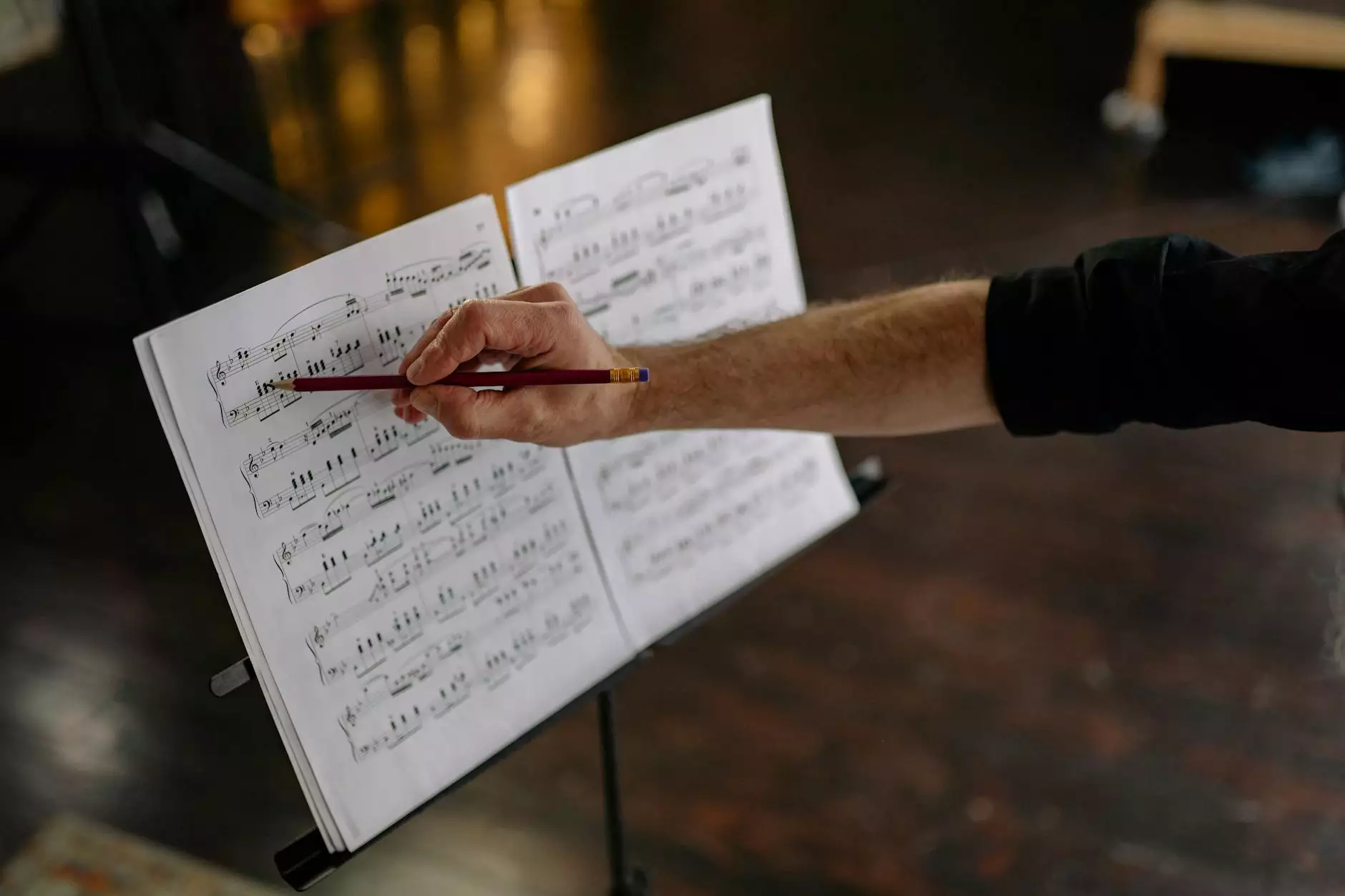
In the rapidly evolving landscape of artificial intelligence and machine learning, bounding boxes have emerged as a pivotal element within the realm of data annotation. At the core of enhancing machine learning algorithms lies the ability to accurately define and label data, which is where bounding boxes play a critical role. In this comprehensive article, we will delve into the significance of bounding boxes in the context of data annotation tools and platforms, exploring how they can drive business success in innovative ways.
Understanding Bounding Boxes
Bounding boxes are rectangular boxes that enclose specific objects of interest within an image or video. By leveraging these visual markers, data annotators can effectively delineate between different entities in a given dataset. This method of annotation is particularly vital in various industries, including autonomous vehicles, retail, healthcare, and more. The need for precision in identifying and classifying objects cannot be overstated, as it directly influences the performance of AI models.
The Role of Bounding Boxes in Data Annotation
Bounding boxes facilitate the process of data annotation by providing a clear and standardized method to segment information within visual data. Each bounding box provides crucial metadata that assists machine learning algorithms in learning from examples. Let’s consider a few applications:
- Autonomous Vehicles: In the automotive industry, vehicles rely heavily on computer vision to navigate their surroundings. Bounding boxes help in identifying other cars, pedestrians, traffic signs, and obstacles, ensuring the safety and efficiency of self-driving technologies.
- Retail and Inventory Management: Retail businesses use bounding boxes to track inventory levels, understand customer behavior, and optimize product placements. This data enables them to make informed decisions that enhance customer experiences.
- Medical Imaging: In healthcare, visual data from imaging technologies like X-rays and MRIs can be annotated with bounding boxes to identify tumors, lesions, and other critical anomalies. This leads to more accurate diagnoses and treatment plans.
Benefits of Using Bounding Boxes in Data Annotation Platforms
When implemented effectively, bounding boxes provide multiple benefits to organizations looking to harness the power of AI:
1. Enhanced Accuracy in Object Recognition
Bounding boxes improve the accuracy of object recognition models. By accurately defining the object of interest, these models can learn from high-quality annotated data, thereby reducing false positives and negatives in future predictions.
2. Streamlined Annotation Process
Many data annotation tools now incorporate bounding box functionality, which simplifies the annotation process for users. By leveraging software that offers smart features such as auto-detection, organizations can significantly reduce the time required for manual annotation.
3. Scalability
As businesses grow, so does their need for large datasets. Bounding boxes allow for scalable annotation practices, enabling companies to annotate vast volumes of images without compromising on quality. This is crucial for machine learning applications that require diverse datasets to perform optimally.
4. Versatility Across Industries
Bounding boxes can be applied across a multitude of industries. Whether for identifying products in retail, monitoring behavior in surveillance footage, or assisting in medical diagnoses, the flexibility of these annotation methods caters to various use cases, making them invaluable.
How Keylabs.ai is Revolutionizing Data Annotation with Bounding Boxes
At Keylabs.ai, we understand the vital role that bounding boxes play in the data annotation landscape. Our state-of-the-art data annotation platform is designed to maximize efficiency and accuracy through innovative features:
- Intuitive Interface: Our user-friendly interface allows for quick annotation of images with bounding boxes. Users can easily adjust box dimensions, colors, and labels to ensure clarity and precision.
- Auto-Annotation Tools: We deploy advanced algorithms that assist in generating bounding boxes, drastically reducing the manual workload and allowing annotators to focus on refining the output.
- Collaboration Features: Teams can collaborate seamlessly on projects, ensuring that all input is consistent and accurate. Notifications and version control help maintain clarity in large teams.
- Quality Assurance Processes: We employ multiple layers of quality checks, ensuring that every bounding box annotation meets the highest standards. This step is crucial for maintaining the integrity of training datasets.
Best Practices for Annotation with Bounding Boxes
To achieve the best results when using bounding boxes, consider the following best practices:
1. Consistency is Key
Establish clear guidelines for bounding box creation to maintain consistency across annotations. This involves defining specific criteria for what constitutes a bounding box for various object types.
2. Regular Training Sessions
Conduct training sessions for annotators to keep them updated on best practices and new features of the annotation tool. An informed annotator will produce higher-quality work, enhancing the dataset’s value.
3. Utilize Quality Control Mechanisms
Implement a robust quality control process that includes reviews and audits of annotated data. By regularly checking against the established guidelines, you ensure that the output consistently meets quality standards.
4. Leverage Technology
Invest in advanced data annotation platforms like those offered by Keylabs.ai that employ machine learning algorithms to assist in bounding box creation. Automation can save time while maintaining or improving accuracy.
Conclusion: Bounding Boxes as a Cornerstone of Data Annotation Success
In conclusion, bounding boxes serve as a fundamental aspect of data annotation that significantly impacts the efficiency and accuracy of machine learning models. With the correct implementation and strategic use of data annotation tools, businesses across industries can leverage these visual markers to train more effective AI systems. Keylabs.ai stands ready to support businesses in realizing the full potential of their data through advanced annotation capabilities.
As the demand for high-quality annotated data continues to grow, investing in a solid data annotation platform that prioritizes bounding boxes can set a business apart from its competitors. Embrace the future of AI with Keylabs.ai, where your data annotation needs are met with precision and innovation.