The Ultimate Guide to Machine Learning Labeling Tools for Data Annotation
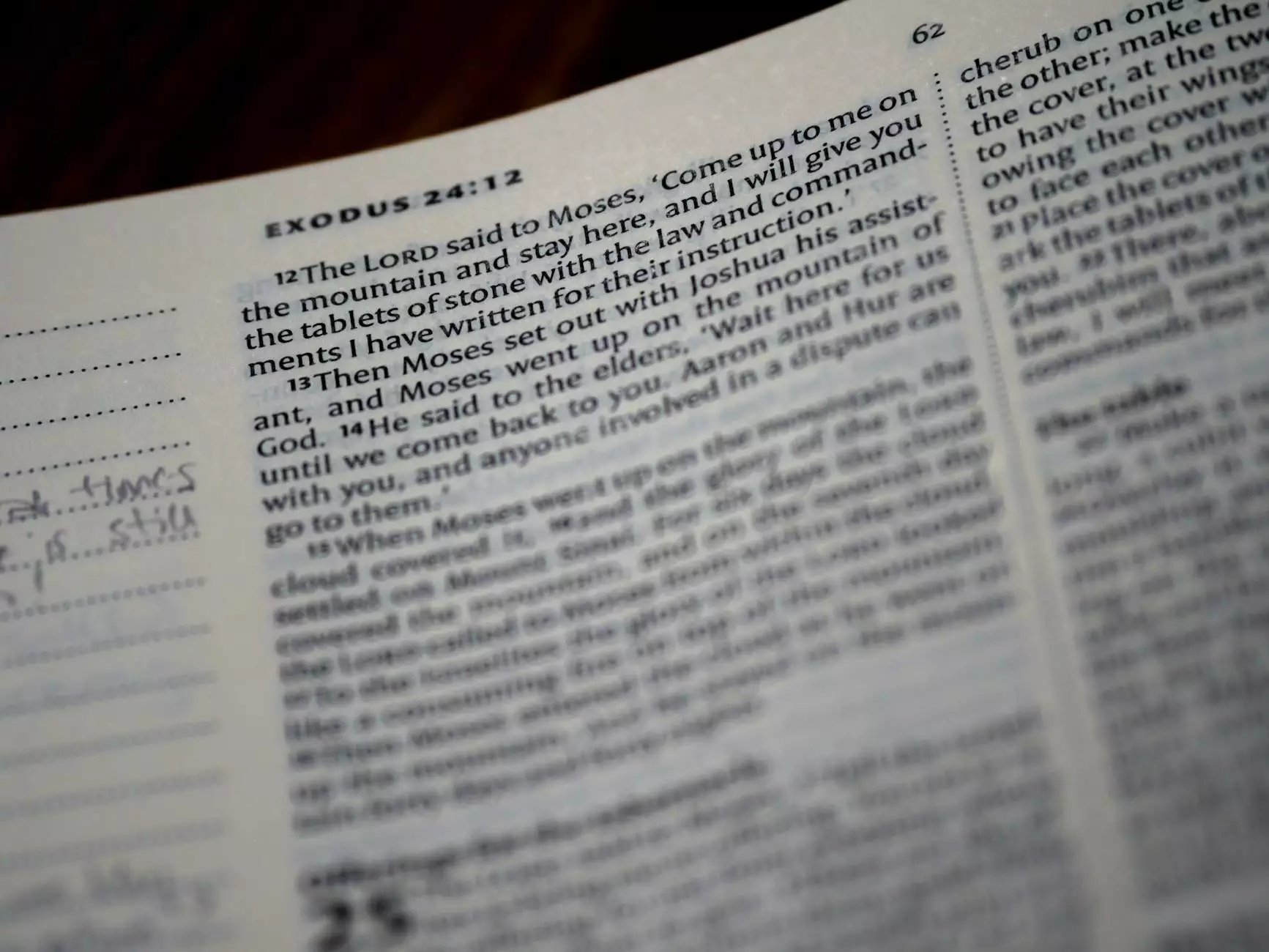
Understanding Data Annotation and Its Importance
In the modern era of artificial intelligence and machine learning, data annotation has become a cornerstone of developing robust machine learning models. This process involves labeling data, which allows algorithms to learn from the inputs they receive and make predictions or decisions based on that data. Accurate and comprehensive labeling is essential for contextual understanding, which ensures that machine learning applications achieve their desired outcomes.
What Are Machine Learning Labeling Tools?
A machine learning labeling tool is software designed to assist in the data annotation process. These tools help users annotate various types of data—such as text, images, audio, and video—accurately and efficiently. By automating parts of this complex task, labeling tools save time and resources while enhancing the quality of the annotated data.
Key Features of Effective Machine Learning Labeling Tools
When searching for a top-tier machine learning labeling tool, there are several essential features that users should consider:
- User-friendly Interface: The tool should have an intuitive and easy-to-navigate interface.
- Customizable Labeling Options: Flexibility in defining labels is crucial to meet diverse project requirements.
- Collaboration Tools: Ability to work in teams, with features allowing seamless communication between annotators.
- Integration Capabilities: The tool should easily integrate with popular data management systems and machine learning platforms.
- Automation Features: Automated processes can enhance efficiency and consistency in data annotation tasks.
- Quality Control Mechanisms: Tools with built-in quality assurance checks ensure higher accuracy in labeling.
The Benefits of Using AI-Powered Data Annotation Tools
AI-powered data annotation is transforming the landscape of how data is labeled across industries. Here are several significant benefits of leveraging such tools:
- Increased Efficiency: Machine learning labeling tools can massively accelerate the annotation process, allowing teams to focus on more complex tasks.
- Consistency and Accuracy: Automated labeling increases the consistency of labels across datasets, leading to higher model reliability.
- Scalability: As project requirements grow, these tools can easily scale, handling vast amounts of data with ease.
- Cost-Effective: Streamlining data annotation can significantly reduce costs, as fewer man-hours are needed for labor-intensive tasks.
- Support for Diverse Data Types: Many tools support various data formats, enhancing their utility across different projects.
Choosing the Right Data Annotation Platform
Selecting the right data annotation platform is pivotal for the success of any machine learning initiative. Developers and data scientists often base their decision on the specific needs of their projects. Here are some factors to consider:
1. Project Specificity
Evaluate whether the platform supports your specific data types and project requirements. Some tools excel in image labeling, while others are better suited for text or audio data.
2. Collaboration Features
For teams working together, look for platforms that facilitate collaboration, allowing multiple users to contribute to projects simultaneously.
3. Support and Community
Good customer support and an active user community can be invaluable resources as you navigate the complexities of data annotation.
4. Customization Options
The ability to customize workflows and labeling formats can significantly enhance your team's productivity.
5. Cost and Licensing
Examine the cost structure of the platform. Some may offer subscription models, while others might have one-time licensing fees. Assess what fits your budget while providing maximum value.
Examples of Popular Machine Learning Labeling Tools
Here are some widely recognized machine learning labeling tools that stand out in the market:
- Labelbox: A comprehensive platform featuring collaboration tools, integrations, and automation capabilities.
- Snorkel: Focuses on programmatic labeling—a unique approach that allows users to generate labeled data without extensive manual effort.
- SuperAnnotate: Known for its user-friendly interface and excellent support for image and video annotation.
- Amazon SageMaker Ground Truth: Provides integrated labeling services with options for human labelers and automation.
- KeyLabs.ai: Offers a robust data annotation platform tailored for diverse industries, focusing on efficiency and quality.
Integrating Machine Learning Labeling Tools into Your Workflow
When considering the implementation of a machine learning labeling tool, it is crucial to seamlessly integrate it into your existing workflows. Here’s a step-by-step approach:
Step 1: Assess Your Current Data Workflows
Identify how data is currently managed, annotated, and utilized within your organization. This assessment will help highlight areas for improvement.
Step 2: Define the Annotation Process
Establish clear guidelines and protocols for data annotation. This includes defining the types of labels and standards to be used to ensure consistency.
Step 3: Train Your Team
Conduct training sessions to familiarize your team with the new tool. Emphasize the importance of accurate labeling to improve model outcomes.
Step 4: Run Pilot Projects
Start with a small-scale pilot project to evaluate how well the tool integrates with your existing systems and workflows before rolling it out on a larger scale.
Step 5: Iterate and Improve
Using feedback from your team, continuously refine the processes and tool usage to enhance the quality of your data annotation.
The Future of Machine Learning Labeling Tools
As artificial intelligence and machine learning continue to advance, the capacity of labeling tools will also evolve. Here are some trends to keep an eye on:
- Increased Automation: Expect more sophisticated AI algorithms that can automate labeling based on patterns and historical data.
- Enhanced Quality Control: Future tools will likely include advanced quality assurance features to maintain high labeling standards.
- Integration with Advanced AI Models: Seamless integration of labeling tools with existing AI models will streamline workflows and improve efficiency.
- Augmented Reality (AR) and Virtual Reality (VR): Innovations in AR and VR will introduce novel ways to annotate data, particularly for image and video.
- Global Collaborations: With the rise of remote work, teams worldwide will collaborate on projects in real-time, necessitating robust online platforms.
Conclusion: The Role of Machine Learning Labeling Tools in Modern Data Annotation
In conclusion, the landscape of machine learning is evolving rapidly, and so is the importance of efficient data annotation through the use of machine learning labeling tools. These tools not only facilitate accurate data labeling but also optimize the workflow for organizations handling vast datasets. By understanding the various aspects of data annotation and selecting the right platform, businesses can ensure they remain competitive in an ever-changing market.
Whether you are a startup or an established entity, integrating powerful data annotation tools like KeyLabs.ai into your operations could be the key to unlocking higher productivity and better model performance. Be proactive about leveraging these resources as you embark on your machine learning journey.